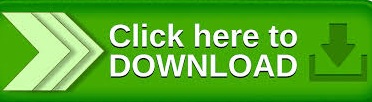
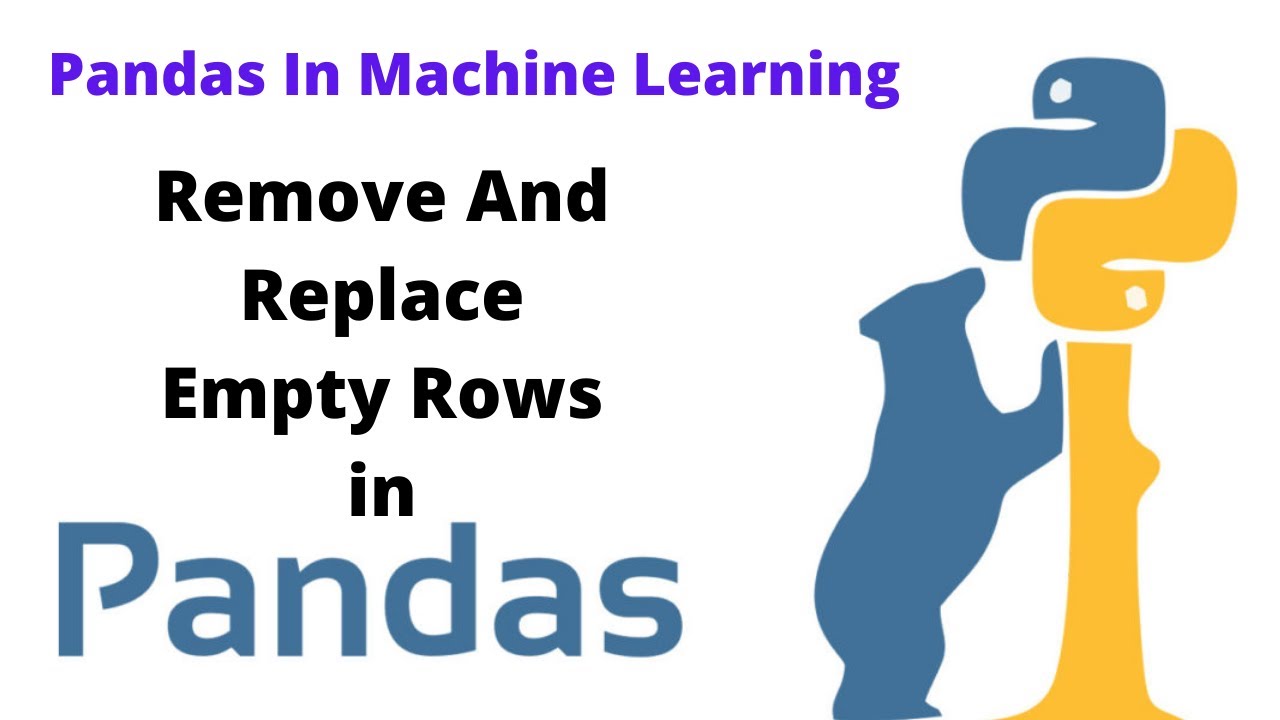
In that study, various aspects, including the time resolution of the employed data, were considered. (2019) reviewed different methods to predict the performance of a PV module. The increasing prominence of machine learning has attracted numerous researchers to this field, whose work has been covered by several recent authoritative reviews.įor example, Akhter et al. In controlling PV systems, machine learning methods have been used for fault detection, but they could also be used to identify improved tracking strategies (Hadipour et al., 2021). Therefore, the application of machine learning has become widespread in both the design and operation of PV infrastructure over recent years (Ni ž eti ć et al., 2021a).ĭuring the design of a PV system, machine learning has been used to identify more accurate ways to optimize the thermal and electrical behavior by addressing phenomena not covered by conventional modeling approaches (Liu et al., 2020). Conventional technologies face several challenges and there has been remarkable progress made in the field of computer science and statistics. In addition, owing to shading, and various other issues, a PV system might operate poorly, requiring appropriate control and fault detection systems (Pan et al., 2020). Locations at which PV systems can function effectively, especially in urban areas, are limited, so that their selection requires careful consideration (Ayan and Toylan, 2021). In a PV system, solar radiation is converted directly into electrical power, while some of the dissipated heat could be recovered and used for a variety of other secondary applications (Dehghan et al., 2021). There are various plans to increase the installed PV capacity in various parts of the world (Akyol et al., 2021). Of the various technologies available to capture solar energy, photovoltaic (PV) systems make a considerable contribution to the overall market (A ğ bulut et al., 2020). This has several advantages, including worldwide availability, as well as the ability to be deployed in a large range of installed capacities (Gagliano et al., 2019). This leads to serious environmental and economic concerns, motivating governments and policy makers to invest in renewable energy systems, especially those based on solar technology (Darbari and Rashidi, 2022). The wider application of machine learning to PV systems could therefore forge a shorter path towards sustainable energy production.Īs the world's population grows, energy production is rising to meet the increasing demand (Abdel-Basset et al., 2021). A key conclusion is that the use of machine learning to analyze PV systems is still in its infancy, with many small-scale PV technologies, such as building integrated photovoltaic thermal systems (BIPV/T), not yet benefiting fully in terms of system efficiency and economic viability. This review also discusses the challenges to and future directions of using machine learning to analyze PV systems. We focus on reviewing the use of machine learning algorithms to predict performance and detect faults, and on discussing how machine learning can help humanity to achieve a cleaner environment in the worldwide drive towards carbon neutrality. Then, the application of machine learning to the analysis of PV systems is discussed.
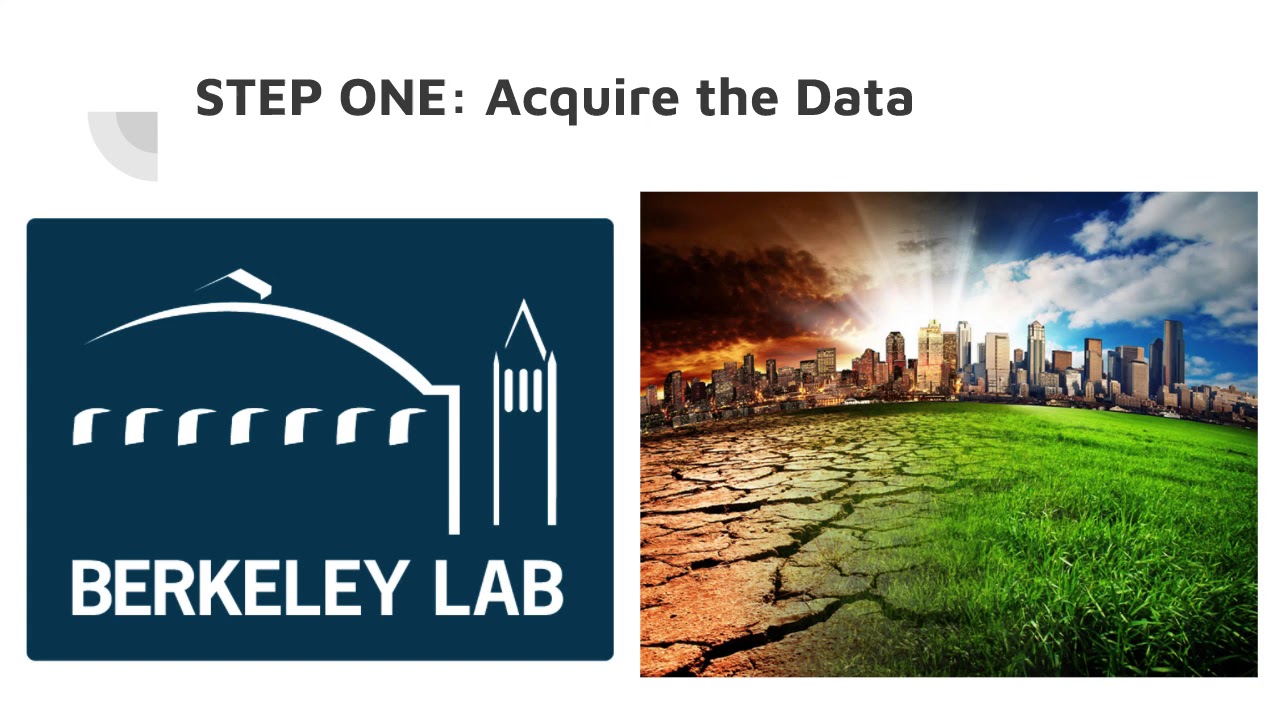
First, conventional methods for modeling PV systems are introduced from both electrical and thermal perspectives.

Against this backdrop, we provide a comprehensive review of machine learning techniques applied to PV systems. In parallel, machine learning has gained prominence because of a combination of factors such as advances in computational hardware, data collection and storage, and data-driven algorithms.

Photovoltaic (PV) technologies are expected to play an increasingly important role in future energy production.
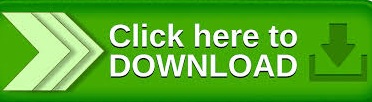